Toronto Machine Learning Society (TMLS) 7th Annual Conference & Expo 2023
Schedule
Mon Jun 12 2023 at 09:00 am to Wed Jun 14 2023 at 08:00 pm
Location
The Carlu | Toronto, ON
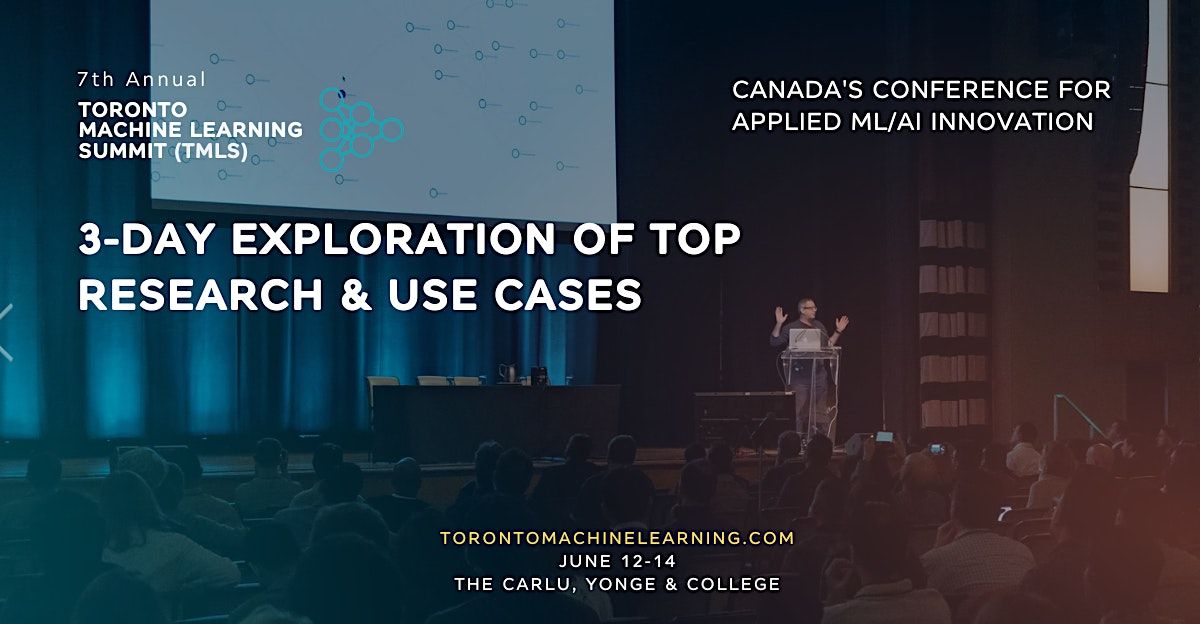
About this Event
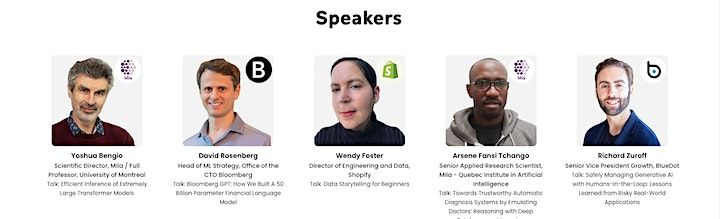
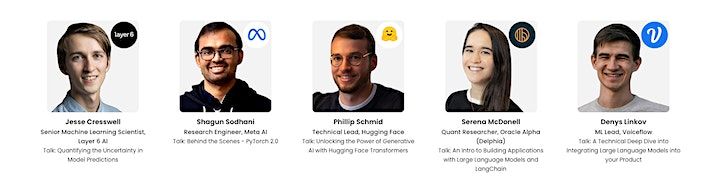
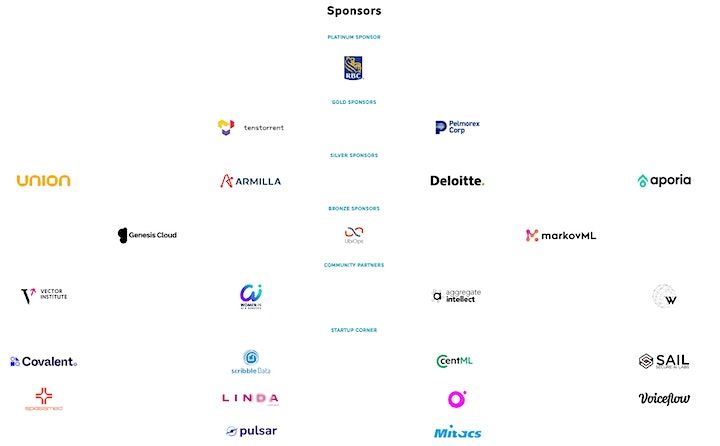
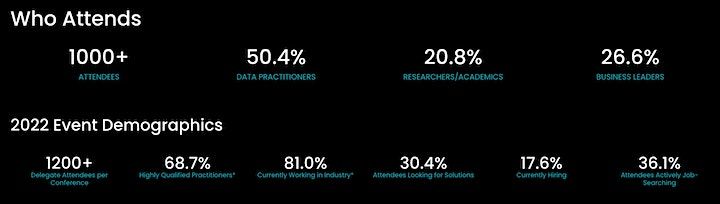
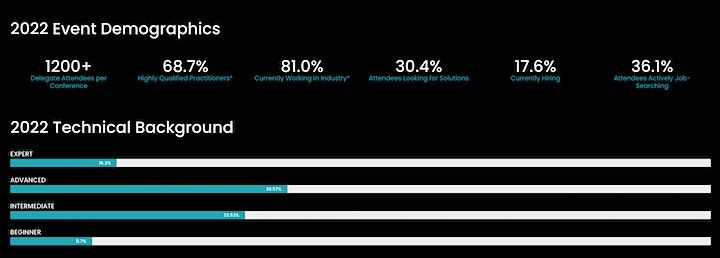
MORE CONFIRMED SPEAKERS TO BE ANNOUNCED SHORTLY.
Join us for an unforgettable experience that happens once annually.
With talks curative by the committee, specifically designed for the growth & development of data practitioners, academics, and ML/AI enthusiasts.
TMLS consists of a community comprised of over 10,000 ML researchers, professionals and entrepreneurs.
We'd like to welcome you to join us in celebrating the top achievements in AI Research, AI companies, and applications within industry.
Expect 2 days of workshops and high-quality networking, food, drinks, workshops, breakouts, keynotes and exhibitors.
Taken from the real-life experiences of our community, the Steering Committee has selected the top applications, achievements from this year's knowledge-areas to highlight across 2 days, and 2 nights.
Come expand your network with machine learning experts and further your own personal & professional development in this exciting and rewarding field.
Included will be:
1,200+ (total) attendees
App-supported pre-event networking.
LEARNINGS:
✅ LangChain workshop
✅ How you can build a generative AI product
✅ A technical deep dive into Integrating Large Language Models into your product
✅ Prompt engineering workshop
✅ Using generative AI open-source models within an enterprise-compliant environment
✅ Explainability & transparency in 2023
and more!
Hands-on Workshops This year workshops will be held on site, June 13-14th
Breakouts and Keynotes on-site
70+ Speakers
AI Career Fair with top 50 AI Start-ups and + job-seekers.
Women in Data Science Ceremony
Poster Session Awards
Social pub networking afterparty
We believe these events should be as accessible as possible and set our ticket passes accordingly
*Please Scroll down for full Program/Abstracts*
The TMLS initiative is dedicated to helping promote the development of AI/ML effectively, and responsibly across all Industries. As well, to help data practitioners, researchers and students fast-track their learning process and develop rewarding careers in the field of ML and AI.
Key Dates:
- June 12th - Virtual workshops (bonus)
- June 13th - In-person talks, networking and parties
- June 14th- In-person talks & Career / Demo Fair
- Technology & Service
- Computer Software
- Banking & Financial Services
- Insurance
- Hospital & Health Care
- Automotive
- Telecommunications
- Environmental Services
- Food & Beverages
- Marketing & Advertising
The TMLS initiative is dedicated to helping promote the development of AI/ML effectively, and responsibly across all Industries. As well, to help data practitioners, researchers and students fast-track their learning process and develop rewarding careers in the field of ML and AI.
Why should I attend the Toronto Machine Learning Society (TMLS) 2023 Annual Conference & Expo:
Developments in the field are happening fast: For practitioners, it's important to stay on top of the latest advances; for business leaders, the implementation of new technology brings specific challenges.
The goal of TMLS is to empower data practitioners, academics, engineers, and business leaders with direct contact to the people that matter most, and the practical information to help advance your projects. For data practitioners, you'll hear how to cut through the noise and find innovative solutions to technical challenges, learning from workshops, case studies, and P2P interactions. Business leaders will learn from the experience of those who have successfully implemented ML/AI and actively manage data teams.
Seminar series content will be practical, non-sponsored, and tailored to our ML ecosystem. TMLS is not a sales pitch - it's a connection to a deep community that is committed to advancing ML/AI and to create and deliver value and exciting careers for Businesses and Individuals.
We're committed to helping you get the most out of the TMLS.
Joining together under one roof will be:
- Machine Learning/deep learning PhDs and researchers
- C-level business leaders
- Industry experts
- Data Engineers, Machine Learning Engineers
- Enterprise innovation labs seeking to grow their teams
- Community and university machine learning groups
Visit: www.torontomachinelearning.com
Steering Committee & Team
Who Attends
Abstracts: What You'll LearnJULY 12TH
Large Neural Nets for Amortized Probabilistic Inference for Highly Multimodal Distributions and Model-Based ML
Yoshua Bengio, Scientific Director / Full Professor, Mila / U. Montreal
About the Speaker: Recognized worldwide as one of the leading experts in artificial intelligence, Yoshua Bengio is most known for his pioneering work in deep learning, earning him the 2018 A.M. Turing Award, “the Nobel Prize of Computing,” with Geoffrey Hinton and Yann LeCun.
He is a Full Professor at Université de Montréal, and the Founder and Scientific Director of Mila – Quebec AI Institute. He co-directs the CIFAR Learning in Machines & Brains program as Senior Fellow and acts as Scientific Director of IVADO.
In 2019, he was awarded the prestigious Killam Prize and in 2022, became the computer scientist with the highest h-index in the world. He is a Fellow of both the Royal Society of London and Canada, Knight of the Legion of Honor of France and Officer of the Order of Canada.
Concerned about the social impact of AI and the objective that AI benefits all, he actively contributed to the Montreal Declaration for the Responsible Development of Artificial Intelligence.
Technical Level: 7
Which talk track does this best fit into? Technical / Research
Abstract: Current large language models and other large-scale neural nets directly fit data, thus learning to imitate its distribution. Is it possible to do better? Consider the possibility, which we claim is actually the typical case, where a world model that captures causal structure in the world would require substantially fewer bits than the code that performs the kinds of inferences that we may desire from that knowledge. For example, the rules of the game of Go or the rules of logical reasoning are fairly compact, whereas inference (e.g., playing Go at Champion level, or being able to discover or prove theorems) may require a lot more computation (i.e., huge neural nets). In fact, exact inference for many problems (in science, computing, or in coming up with Bayesian posteriors) is often intractable and so we resort to approximate solutions. With amortized probabilistic inference, we expend computation upfront to train a neural net that learns to answer questions (for example sample latent variables or parameters) in a way that is as consistent as possible with the world model, making run-time inference quick. This also makes it possible to exploit the generalization ability of the approximate inference generative learner to guess where good answers (e.g., modes of a highly multimodal posterior distribution) might be. It also allows to decouple the capacity needed for inference from the capacity needed to describe the world model. This is unlike current state-of-the-art in deep learning, where inference and world model are confounded, yielding overfitting of the world knowledge and underfitting of the inference machine. We have recently introduced a novel framework for achieving this kind of model-based ML, with generative flow networks (or GFlowNets), which have relations to reinforcement learning, variational inference and generative models. We'll highlight some of the advances achieved with GFlowNets and close with our research programme to exploit such probabilistic inference machinery to incorporate in ML inductive biases inspired by high-level human cognition and build AI systems that focus on understanding the world in a Bayesian and causal way and generating probabilistically truthful statements.
Explainable AI in Finance: Present and Future Challenges
Freddy Lecue, AI Research Director, JPMorgan Chase & co.
About the Speaker: Dr Freddy Lecue (PhD 2008, Habilitation 2015) is AI Research Director at J.P.Morgan in New York. He is also a research associate at Inria, in WIMMICS, Sophia Antipolis - France. Before joining J.P.Morgan he was the Chief Artificial Intelligence (AI) Scientist at CortAIx (Centre of Research & Technology in Artificial Intelligence eXpertise) @Thales in Montreal, Canada from 2019 till 2022. Before his leadership role at the new R&T lab of Thales dedicated to AI, he was AI R&D lead at Accenture Technology Labs, Dublin - Ireland. Prior joining Accenture he was a research scientist, lead investigator in large scale reasoning systems at IBM Research from 2011 to 2016 Before his AI R&D lead role, Freddy was a principal scientist and research manager in Artificial Intelligent systems, systems combining learning and reasoning capabilities, in Accenture Technology Labs, Dublin - Ireland. Before joining Accenture in January 2016, he was a research scientist and lead investigator in large scale reasoning systems at IBM Research - Ireland.
Which talk track does this best fit into?: Technical / Research
Technical level of your talk? 5
Abstract: Financial institutions require a deep understanding of the rationale behind critical decisions, and particularly the predictions made by machine learning models. This is particularly true in high-stake decision-making such as loan underwriting and risk management. This has a led to a growing interest in developing interpretable and explainable Artificial Intelligence (XAI) techniques for machine learning models that can provide insights into the model's decision-making progress. The development of these models will be crucial for the widespread adoption of machine learning (including deep learning) in finance and other regulated industries. This presentation will first give an overview on the recent progress of adopting and enriching XAI techniques in finance, and then review recent challenges where XAI techniques would need to be pushed further to embed the latest advancement of AI techniques including Large Language Models.
Challenges and Learnings of Machine Learning at scale
Raheleh Givehchi, Lead Data Scientist, Pelmorex Corp. (The Weather Network)
Hicham Benzamane, Team Lead, Data Engineering Insights, Pelmorex Corp. (The Weather Network)
About the Speaker:
Raheleh Givehchi - Lead Data Scientist:
I am a lead data scientist with several years of experience in data science and machine learning. I specialize in delivering valuable insights through data analytics and advanced data-driven methods. Currently, I am working on the Weather Insight Platform (WIP) to provide data-driven solutions to weather-related challenges.
Hicham Benzamane - Team Lead, Data Engineering Insights:
- Multiple years working experience in software development and Data engineering.
- Now leading a team of software and data engineers to leverage data and ML to empower Pelmorex customers with cutting edge insights.
Which talk track does this best fit into?: Virtual Workshop
Technical level of your talk? 5
What you'll learn: Real world Data optimization techniques for training and prediction and Scale techniques using Cloud infrastructure
Pre-requisite Knowledge: Basics on Machine Learning and Basics on Cloud Computing
Abstract of talk: How to train millions of models while keeping cost very low and predict on daily basis with the highest performance.
Fine-tuning Language Models with Declarative ML Orchestration
Niels Bantilan , Chief Machine Learning Engineer, Union.AI
About the Speaker: Niels is the Chief Machine Learning Engineer at Union.ai, and core maintainer of Flyte, an open source workflow orchestration tool, author of UnionML, an MLOps framework for machine learning microservices, and creator of Pandera, a statistical typing and data testing tool for scientific data containers. His mission is to help data science and machine learning practitioners be more productive.
He has a Masters in Public Health with a specialization in sociomedical science and public health informatics, and prior to that a background in developmental biology and immunology. His research interests include reinforcement learning, AutoML, creative machine learning, and fairness, accountability, and transparency in automated systems.
Which talk track does this best fit into?: Virtual Workshop
Technical level of your talk? 4
What you'll learn: This workshop has two main learning goals. First, attendees will learn the main concepts behind Flyte, a workflow orchestrator for data and machine learning. Many of these concepts are orchestrator-agnostic, such as containerization for reproducibility, declarative infrastructure, and type-safety. Secondly, they will also learn how to leverage the latest deep learning frameworks that optimize memory and compute resources required to fine-tune language models in the most economical way.
Pre-requisite Knowledge: Intermediate Python, working knowledge of Docker, and intermediate knowledge of machine learning.
Abstract of talk: The use of Language Models (LMs) has become more widespread in recent years, thanks in part to the broader accessibility of datasets and the ML frameworks needed to facilitate the training of these models. Many of these models are large – hence the terminology of Large Language Models (LLMs) – and serve as so-called foundation models, which are trained by organizations with the compute resources to train them. These foundation models, in turn, can be fine-tuned by the broader machine learning community for specific use cases, perhaps on proprietary data. One of the barriers that make fine-tuning these models is infrastructure: even with cloud tools like Google Colab and the wider availability of consumer-grade GPUs, putting together a runtime environment to fine-tune these models is still a major challenge. This workshop will give attendees hands-on experience on how to use Flyte to declaratively specify infrastructure so that they can configure training jobs to run on the required compute resources to fine-tune LMs on their own data.
Deploying generative AI models: best practices and an interactive example
Anouk Dutrée, Product Owner, UbiOps
About the Speaker: Anouk is a Product Owner at UbiOps. She studied Nanobiology and Computer Science at the Delft University of Technology, which spiked her interest in Machine Learning. Next to her role at UbiOps, she also frequently writes for Towards Data Science about various MLOps topics, she co-hosts the biggest Dutch data podcast, de Dataloog, and she recently rounded up a Master’s in Game Development. Her efforts in tech have been awarded twice with the Tech 500 award (T500), in both 2020 and 2021.
Which talk track does this best fit into? Virtual Workshop
Technical level of your talk? 4
What you'll learn: Deployment at scale doesn't have to be difficult. Participants will learn how to deploy a generative AI model to the cloud themselves, and how to make sure it runs with the right resources (CPU,GPU,IPU etc.).
Pre-requisite Knowledge: Python knowledge and a basic understanding of what computer vision is.
Abstract of talk: Generative AI models are all the hype nowadays, but how do you actually deploy them in a scalable way? In this talk we will discuss best practices when moving models to production, as well as show an interactive example of how to deploy one using UbiOps. UbiOps is a serverless and cloud agnostic platform for AI & ML models, built to help data science teams run and scale models in production. We will pay special attention to typical hurdles encountered in deploying (generative) AI models at scale. Python knowledge is all you need for following along!
Making GenAI Safe, Trustworthy and Fit-for-purpose with Auto Alignment
Rahm Hafiz, CTO and Co-Founder, Armilla AI
Dan Adamson,CEO and Co-founder, Armilla AI
About the Speaker: Rahm Hafiz is the co-founder and CTO of Armilla AI, a company helping institutions testing and building more fair, safe, trustworthy, and useful AI. In the past, Rahm headed AI initiatives at Outside IQ and Exiger where he worked with global financial institutions, government and regulatory bodies to bring innovative AI into reality. Rahm’s PhD work offers an efficient and modular framework for syntactic and semantic analyses and understanding of natural language by addressing some long standing NLP problems including correct processing of ambiguity. Rahm’s research on NLP has been published in over 10 reputable journals and conferences.
Dan Adamson is the Co-Founder and CEO of Armilla.AI, a company helping institutions testing and building more fair, safe, trustworthy, and useful AI. Previously, he founded and served as OutsideIQ’s CEO, since its inception in 2010 until its acquisition in 2017 by Exiger, where he remained as their President overseeing product and cognitive computing research. Dan also previously served as Chief Architect at Medstory, a vertical search start-up acquired by Microsoft. He is an expert on vertical search, investigative use of big data and cognitive computing with more than 15 years in the industry. He holds several search algorithm and cognitive computing patents, has been named among the most influential “must-see” thought leaders in AI and FinTech, in addition to being a recipient of numerous academic awards and holding a Master of Science degree from U.C. Berkeley.
Which talk track does this best fit into?: Virtual Workshop
Technical level of your talk? 4
What you'll learn: By the end of the workshop, participants will understand common shortcomings of Gen AI, how to use a self-governing alignment technology to overcome those shortcomings and to make GenAI useful for user specific tasks.
Pre-requisite Knowledge: Concepts of large language models, text 2 image models.
Abstract of talk: We discuss a new alignment templating technology that can be used to enhance the fairness, robustness and safety of the generative AI by understanding the expected behaviour of the model, measuring where the model is underperforming with synthetic test data, and iteratively improving the model with minimal humans in the loop fine-tuning approach. This alignment platform can be used for use case specific tasks including coercing a generative model to be more fair towards under represented groups, less toxic and less misogynistic, more leaning towards a desired political viewpoints, to use specific tone while generating answers for customer service applications, to preserve PII, to guard models from generating potentially harmful responses etc. Our alignment technology can interact with users as needed to adjudicate critical decision points to guide its intention-understanding, data generation, testing and tuning capabilities to be more contextual.
Creating an ML model as a business user
Kevin Laven, Energy & Resource Leader, Deloitte
Lynn Luo, Senior Manager, Deloitte
Alex Gobolos, Solutions Engineer, Dataiku
About the Speaker: Kevin Laven leads the Energy, Resources, and Industrial sector team within Deloitte Canada’s Artificial Intelligence practice. Kevin’s AI experience started in 2003 with a Masters degree at the University of Toronto Machine Learning Lab, and includes dozens of AI models for ER&I applications.
Alex is a Solutions Engineer at Dataiku. He works with customers to get value from all things data and analytics, from data access and exploration, to machine learning and AI. Alex has worked in the analytics space for the last 15+ years including project delivery, consulting, and solutions engineering across industries such as banking and insurance, healthcare, and manufacturing.
Which talk track does this best fit into?: Virtual Workshop
Technical level of your talk? 4
What you'll learn: High-level steps on creating an ML model as a business user
Pre-requisite Knowledge: Basic knowledge of ML
Abstract of talk: Opportunity for business users and executives to be exposed to the following topics: How to validate use cases, Approaches to building models, Business case for deployment
Building an end-to-end web application integrated with Microsoft's Semantic Kernel and a Large Language Model
Chinmay Sheth, Senior Machine Learning Engineer, Royal Bank of Canada (RBC)
Colleen Gilhuly, Royal Bank of Canada (RBC)
Haleh Shahzad, Director, Data Science, Royal Bank of Canada (RBC)
About the Speaker: Chinmay Sheth is a Senior Machine Learning Engineer at the Royal Bank of Canada and is completing his MSc in Computer Science at McMaster University. His responsibilities include providing support for MLOps tooling, promoting ML models to production, and developing production-grade data pipelines.
Colleen TBD
Haleh is a Director, Data Science at RBC with 9+ years of experience in AI (Deep learning, machine learning), software development and advanced analytics. She is currently leading AI initiatives for cutting-edge solutions to maximize the impact of data across organization at RBC. She is also an instructor in the school of continuing studies at York University. Haleh has a Ph.D. degree in Electrical and Computer Engineering from McMaster university where she was also part of the sessional faculty in the Electrical and computer engineering department.
Which talk track does this best fit into?: Virtual Workshop
Technical level of your talk? 5
What you'll learn: - Learn the basics of LLMs, and their applications/impacts in industry
- Hands-on experience with Semantic Kernel’s skills, planner, memories, and chains
- You will be able to build your own web app with an LLM integrated into the backend
Abstract of talk: Large language models (LLMs) such as ChatGPT are able to interpret text input and generate human-like responses. Many individuals and companies are excited to use this technology, but integration remains a questionmark. Applications using LLMs are also limited by their tendency to invent information and give unpredictable answers. Microsoft’s Semantic Kernel is an open source, lightweight SDK which enables fast integration of LLMs into a wide range of applications. It also enhances the power of LLMs with a structured approach to responses and the ability to refer to external sources of truth. In this workshop, we will give a crash-course on Microsoft Semantic Kernel and demonstrate how to create a simple web app that harnesses the power of LLMs from OpenAI. This workshop will be hands on so please come prepared with an OpenAI API key.
An open-source approach to build guardrails for deploying ML models in healthcare.
Amrit Kishnan, Senior Applied Machine Learning Specialist,Vector Institute
About the Speaker: Amrit is a Senior Applied ML Specialist at the Vector Institute, currently working on building tools for enabling ML deployment for healthcare. Amrit is passionate about Healthcare ML, Robotics, Open-Source and Software Engineering for productionizing ML systems.
Which talk track does this best fit into?: Virtual Workshop
Technical level of your talk? 5
What you'll learn: You will learn how to use an open-source toolkit to develop ML models on health data, with a focus on deployment and workflow integration.
Pre-requisite Knowledge: Clinical data, ML, Software development, Open-source
Abstract of talk: The use of ML in healthcare applications is rising steadily. Deployment of these systems requires a responsible approach, and regulation is lagging behind. At the Vector Institute, in strong collaboration with our stakeholders, we are building an open-source software framework to address this gap. Specifically, we are focussing on rigorous evaluation and monitoring of the ML system across patient sub-populations. We will show how we can generate evaluation and monitoring reports for end-users, using two use cases. Additionally, we will also discuss challenges in implementing monitoring sub-systems in healthcare settings.
JULY 13TH
BloombergGPT: How we built a 50 billion parameter financial language model
David Rosenberg, Head of ML Strategy, Office of the CTO, Bloomberg
About the Speaker: David Rosenberg leads the Machine Learning Strategy team in the Office of the CTO at Bloomberg. He is also an adjunct associate professor at the Center for Data Science at New York University, where he has repeatedly received NYU’s Center for Data Science “Professor of the Year” award. Previously, he was Chief Scientist at Sense Networks, a location data analytics and mobile advertising company, and served as scientific adviser to Discovereads, a book recommendation company first acquired by Goodreads and later Amazon. He received his Ph.D. in statistics from UC Berkeley, where he worked on statistical learning theory and natural language processing. David received a Master of Science in applied mathematics, with a focus on computer science, from Harvard University, and a Bachelor of Science in mathematics from Yale University. He is currently based in Toronto.
Which talk track does this best fit into? Technical / Research
Who is this presentation for? Senior Business Executives, Product Managers, Data Scientists/ ML Engineers and High-level Researchers
What you’ll learn: You'll learn about the challenges that are often faced when designing and training LLMs, as well as some approaches to address these challenges. You'll also see how domain-specific datasets can benefit LLMs.
Technical Level: 5
Are there any industries (in particular) that are relevant for this talk? Banking & Financial Services
Pre-requisite Knowledge: This talk should be accessible to anybody who has some experience training deep learning models. It will be of particular interest to individuals who are considering building such a large language model, as well as those who are planning to do so.
What are the main core message (learning) you want attendees to take away from this talk? There's a lot involved with training a large language model.
What is unique about this speech, from other speeches given on the topic? I was part of the team that built BloombergGPT and co-authored the paper.
Abstract: We will present BloombergGPT, a 50 billion parameter language model, purpose-built for finance and trained on a uniquely balanced mix of standard general-purpose datasets and a diverse array of financial documents from the Bloomberg archives. Building a large language model (LLM) is a costly and time-intensive endeavor. To reduce risk, we adhered closely to model designs and training strategies from recent successful models, such as OPT and BLOOM. Nevertheless, we faced numerous challenges during the training process, including loss spikes, unexpected parameter drifts, and performance plateaus. In this talk, we will discuss these hurdles and our responses, which included a complete training restart after weeks of effort. Our persistence paid off: BloombergGPT ultimately outperformed existing models on financial tasks by significant margins, while maintaining competitive performance on general LLM benchmarks. We will also provide several examples illustrating how BloombergGPT stands apart from general-purpose models. Our goal is to provide valuable insights into the specific challenges encountered when building LLMs and to offer guidance for those debating whether to embark on their own LLM journey, as well as for those who are already determined to do so.
Can you suggest 2-3 topics for post-discussion? 1) Challenges building LLMs 2) Optimizing the cloud for training LLMs
Profiling ML workloads for energy footprint
Main Speaker: Akbar Nurlybayev, Cofounder, CentML
Co- speakers: Michael Shin, Senior Software Development Engineer (Senior SDE),CentML
Yubo Gao, Research Software Development Engineer (RSDE) at CentML and Ph.D student at UofT, CentML
John Calderon, Software Engineer, CentML
About the Speaker: Akbar - Co-founder of CentML, where we accelerate ML workloads by optimizing models to run efficiently on GPUs without sacrificing model accuracy. Prior to CentML, I lead a data organization at KAR Global, through digital transformation and modernization of their data platform.
Michael - Michael is helping CentML to build our open-source machine learning tools, that help ML practitioners to performance profile their models, figure out appropriate deployment targets and measure CO2 impact.
Yubo - Yubo is responsible for the research aspects of CentML's open source tools for ML practitioners that let users profile their models, figure out appropriate deployment targets and measure CO2 impact.
John - Software Engineer at CentML working on machine learning profiling tools
Which talk track does this best fit into?: Workshop
Technical level of your talk? 4
What you'll learn: As described in the abstract, given that no one is thinking about profiling for energy and CO2 impact in ML world, the available tools are not widely used and as a result not very useful.
Abstract of talk: It’s no secret that deep learning requires a lot of compute power for both training and inference. Often businesses evaluate the value of machine learning at enterprise from cost perspective, i.e. people (salaries) and infrastructure (cloud spending). Increasingly a lot of organizations adding an environmental mandates to their business goals, such as reducing CO2 emissions from their compute workloads.
In this workshop we are responding to this need. We will be building on top of the previous workshop on optimizing machine learning workloads at TMLS 2022 and demonstrate the reduction of environmental cost of the optimized workloads versus status quo. We will do a survey of the landscape of tools available now, and show some of the free open-sourced tools we built at CentML for energy profiling.
A Technical Deep Dive into Integrating Large Language Models into Your Product
Denys Linkov, ML Lead, Voiceflow
About the Speaker: Denys is the ML lead at Voiceflow focused on building the ML platform and data science offerings. His focus is on realtime NLP systems that help Voiceflow's 60+ enterprise customers build better conversational assistants. His role alternates between product management, ML research and ML platform building. Previously he worked at large global bank as a senior cloud architect.
Which talk track does this best fit into? Workshop
Who is this presentation for? Senior Business Executives, Product Managers, Data Scientists/ ML Engineers and High-level Researchers, Product Managers, Data Scientists/ ML Engineers, ML Engineers
What you’ll learn: A real life use case with challenges and successes in a rapidly evolving space.
Technical Level: 6
Are there any industries (in particular) that are relevant for this talk? All
Pre-requisite Knowledge: Large language models basic concepts, general ML and programming terminology
What is unique about this speech, from other speeches given on the topic? We've achieved good initial uptake in our generative features in a space (conversational ai) that has been disrupted by chat gpt. Many teams are hesitant to share their approach since it is seen as a competitive advantage.
Abstract: You see a demo of a Large Language Model (LLM) and want to integrate it into your product, but what's next? In this workshop we'll go through both the product planning and technical implementation of integrating a LLM into your product. This will include performance monitoring, integrations, testing, fine tuning, and the various strategies we experimented with at Voiceflow. We'll share some of the challenges and solutions we faced, and each module will feature a notebook to follow along with.
Can you suggest 2-3 topics for post-discussion? How to rapidly prototype new ideas, what technology stack we used, how do we approach research vs application.
Quantifying the Uncertainty in Model Predictions
Jesse Cresswell, Sr. Machine Learning Scientist, Layer 6 AI / TD
About the Speaker: Jesse is a Senior Machine Learning Scientist at Layer 6 AI within TD, and is the Team Lead for Credit Risk. His applied work centers on building machine learning models in high risk and highly regulated domains. Jesse leads research on trustworthy AI and privacy enhancing technologies for machine learning.
Which talk track does this best fit into? Technical / Research
Who is this presentation for? Senior Business Executives, Product Managers, Data Scientists/ ML Engineers and High-level Researchers
What you’ll learn: Conformal prediction is a mathematical/statistical procedure which may be difficult to understand for less technical audience members. In my talk I will cut through the mathematics to provide the intuition of conformal prediction using visual aids and real-world examples. The audience will take away several settings where they ca immediately apply the technique.
Technical Level: 6
Are there any industries (in particular) that are relevant for this talk? Banking & Financial Services, Computer Software, Hospital & Health Care, Insurance, Marketing & Advertising
Pre-requisite Knowledge: General understanding of predictive modelling, basic statistics.
What are the main core message (learning) you want attendees to take away from this talk? Most models do not specify how certain they are about their outputs, but conformal prediction can quantify the uncertainty of any model in a statistically rigorous way, and requires only a few extra lines of code.
What is unique about this speech, from other speeches given on the topic? This topic has not been seen before at TMLS. I will not shy away from the mathematical understanding, since that is a main benefit of the approach, but my focus will be on providing the intuition first, visually. As a lead scientist at Layer 6 AI @ TD, I have personally used conformal prediction in real-world financial modelling applications and can provide the audience guidance on the best practices.
Abstract: Neural networks are infamous for making wrong predictions with high confidence. Ideally, when a model encounters difficult inputs, or inputs unlike data it saw during training, it should signal to the user that it is unconfident about the prediction. Better yet, the model could offer alternative predictions when it is unsure about its best guess. Conformal prediction is a general purpose method for quantifying the uncertainty in a model's predictions, and generating alternative outputs. It is versatile, not requiring assumptions on the model and being applicable to classification and regression alike. It is statistically rigorous, providing a mathematical guarantee on model confidence. And, it is simple, involving an easy three-step procedure that can be implemented in 3-5 lines of code. In this talk I will introduce conformal prediction and the intuition behind it, along with examples of how it can be applied in real-world usecases.
Can you suggest 2-3 topics for post-discussion? What other methods are used for uncertainty quantification? What should be done when a model signals it has low confidence? How can we train models so that their confidence levels are more realistic?
Demystifying Large Language Models: ChatGPT, GPT-4, and the Future of AI Communication
Alex Olson, Senior Research Associate, University of Toronto, CARTE
Somayeh Sadat, Assistant Director, University of Toronto, CARTE
About the Speaker: At CARTE, Alex collaborates with faculty, industry partners, and students to bring the latest advances in AI to a broad audience. He has contributed to machine learning-based research in a wide variety of fields, and runs training for learners at every level.
Somayeh Sadat is the assistant director at the Centre for Analytics and Artificial Intelligence Engineering (CARTE), University of Toronto. In this role, she facilitates and oversees partnerships with industry and government to collaboratively conduct state-of-the-art research and translate and commercialize effective solutions in analytics and artificial intelligence, where applicable with leveraged funds. She also helps nurture the next generation of engineering talent through facilitating experiential learning opportunities with external partners. Somayeh holds a Ph.D. from the University of Toronto, and has over fifteen years of experience in research and education, business development, and consulting.
Which talk track does this best fit into? Case Study
Who is this presentation for? Senior Business Executives, Product Managers, Data Scientists/ ML Engineers and High-level Researchers
What you’ll learn: I have experience giving this talk to a completely non-technical audience, for which I've had great feedback. The aim of this talk is for people to walk away feeling like they actually understand the functionality of LLMs, without needing a tchnical background.
Technical Level: 1
Are there any industries (in particular) that are relevant for this talk? All
Pre-requisite Knowledge: Minimal
What is unique about this speech, from other speeches given on the topic? I have experience explaining complex AI concepts to a completely non-technical audience.
Abstract: The rapid advancements in artificial intelligence (AI) have given rise to a new era of large language models (LLMs) such as ChatGPT and GPT-4. These sophisticated AI systems are capable of understanding and generating human-like text, making them invaluable tools for communication and problem-solving. However, the complexity of these models often leaves the general public feeling overwhelmed and unsure of their implications. This talk aims to demystify LLMs, explaining their inner workings in an accessible manner, while highlighting their potential impact on our society.
Automated Inspection for Rail Cars Using Computer Vision and Machine Learning
Ashley Varghese, Data Scientist, Canadian National Railways (CN)
About the Speaker: Ashley is a data scientist at Canadian National Railway. She works in the automated inspection program overseeing the development and retraining aspects for the inspection of rail cars. She has over 12 years of research experience in computer vision and deep learning. Her research papers were published in multiple international conferences and journals. She has previously worked as an AI Scientist with Qii.AI and as a researcher with TCS Innovation Lab. She holds an MTech in Computer Science from the International Institute of Information Technology, Bangalore.
Which talk track does this best fit into? Case Study
Who is this presentation for? Senior Business Executives, Product Managers, Data Scientists/ ML Engineers and High-level Researchers, Product Managers, Data Scientists/ ML Engineers, ML Engineers, Researchers
What you’ll learn: This talk provides insight into how computer vision and machine learning are applied in the railroad domain for the inspection of rail cars. It includes the challenges, strategies, and practical considerations that are associated with developing an ML-based automated inspection pipeline for a real-world, safety-critical application.
Technical Level: 4
Are there any industries (in particular) that are relevant for this talk? Railroad
Pre-requisite Knowledge: Fundamental understanding of machine learning and computer vision
What are the main core message (learning) you want attendees to take away from this talk? This talk provides insight into how computer vision and machine learning are applied in the railroad domain for the inspection of rail cars. It includes the challenges, strategies, and practical considerations that are associated with developing an ML-based automated inspection pipeline for a real-world, safety-critical application.
What is unique about this speech, from other speeches given on the topic? This talk is based on my first-hand experience in developing and deploying ML pipelines for a real-world inspection program. This provides insight into real-world data, the challenges associated with training a robust model, and evaluate the performance of the model in production, and improve the model performance with retraining.
Abstract: CN is one of the organizations which realized the importance of AI very early and adopted some of the latest technologies in railroad operations. We at CN have been evaluating and adopting some of the latest approaches to carry out automated inspections of railcars that improve overall operational efficiency. The automated inspection of the railcars is achieved by leveraging computer vision and machine learning techniques. For automated inspection of railcars, the train passes through the portals which have equipped with two generations of cameras and are positioned to capture all sides of the railcar including the undercarriage. Once these images are captured, they are sent to the inference engine to detect the defects. However, developing machine learning pipelines and training robust models comes with its own challenges. In this talk, I would be covering how computer vision and machine learning techniques are used for developing some of the use cases, and various challenges associated with developing these machine learning pipelines. One of the key challenges is selecting the right data for training which must be representative of the actual data from the portal. Self-supervised learning-based techniques are adopted to identify the unique samples from the pool of unlabeled datasets. Another challenge associated with the model performance is image quality. The quality of the image captured at the portals is affected by different weather and lighting conditions. In addition to these, there are several other challenges such as subjectivity in defect classification, lack of samples for the defective class and imbalanced datasets, etc. I will pick one of the use cases we developed and then go over discussing some challenges faced and how we tackled these challenges to improve the performance of the use cases. By choosing the right data and computer vision approaches, it is possible to develop effective solutions for the automated inspection of cars.
Can you suggest 2-3 topics for post-discussion? The challenges associated with real-world inspection scenarios, data-centric AI approaches, and building ML pipelines for rail car inspection.
How Canada's Research Institutes are Approaching Generative AI
Cameron Schuler, Chief Commercialization Officer & VP, Industry Innovation Vector Institute
Kirk Rockwell, VP Public Strategy, Amii
Michel Dubois, Director, AI Activist, Mila
About the Speaker: As Director, AI Activation at Mila, the Quebec Artificial Intelligence Institute, Michel Dubois actively participates in the development of AI for the benefit of all. He holds a master's degree in mathematics and is currently a PhD candidate in engineering (machine learning). He is also the author of a patent on the mathematical optimization of high-bandwidth signal switching. Over the past 28 years, Michel Dubois has consolidated his experience in several aspects of machine learning and artificial intelligence. Before joining Mila, Michel Dubois held the role of Associate Partner at IBM, and, in his previous role, he was Vice President of Artificial Intelligence at Newtrax, a Montreal start-up that quickly experienced international success, reaching an average annual revenue growth of 80% and opening offices in Santiago, Perth, Moscow and London.
Cameron Schuler is the Chief Commercialization Officer & Vice President, Industry Innovation at the Vector Institute. He is the former Executive Director of Amii, where, for 8 years, he led one of the top-ranked Machine Learning and AI groups in the world. Cameron’s multifaceted career has covered finance, business & product development, consumer products, IT and general management from start-ups to mature companies. His industry experience includes Alternative Energy, Banking, Consumer Products, Information Technology (Consumer and Enterprise), Investment Sales and Trading, Life Sciences, Manufacturing, Medical Devices, Oil & Gas, and Oil & Gas Services. Roles have included COO, CFO, President and CEO and he was COO & CFO of a food manufacturer whose products lead to sales of over 250 million units.
Kirk Rockwell is a project and operations manager with more than 20 years of experience managing technology and innovation initiatives, across a broad range of sectors in partnership with academia, all levels of government, and some of Canada’s largest companies. Within an arm's length government agency, he had responsibility for investment of public funds into research and innovation projects across multiple sectors including energy, clean tech, agriculture and health, utilizing technologies like AI and Machine Learning, Nanotechnology and Genomics. He has experience with numerous public/private partnerships (P3s) including membership on advisory and governance boards where the stakeholders include large multinational corporations, the governments of Alberta and Canada, universities, and other small or medium-sized companies. He has a Diploma in Environmental Technologies, a Bachelor of Science Degree in Environmental Sciences, and a Master’s of Business Administration Degree with a specialization in Innovation and Entrepreneurship. Kirk is currently the VP, Public Strategy and Gants at the Alberta Machine Intelligence Institute (Amii) in Edmonton, Alberta, Canada. He resides in Edmonton with his wife and two daughters.
Which talk track does this best fit into? Technical / Research
Technical Level: 4
Are there any industries (in particular) that are relevant for this talk? Automotive, Banking & Financial Services, Computer Software, Environmental Services, Food & Beverages, Hospital & Health Care, Information Technology & Service, Insurance, Marketing & Advertising, Telecommunications.
What are the main core message (learning) you want attendees to take away from this talk? (Panel discussion)
What is unique about this speech, from other speeches given on the topic? (Panel discussion)
Abstract: (Panel discussion)
Using Deep Learning to Build Offer Recommendation System in Fast Food Industry
Gavin Fan, Senior Manager, Head of Data Science & Machine Learning - Tim Hortons
About the Speaker: Gavin is the machine learning expert with multiple years working experience with the scope ranging from CRM to large complicated recommender system and NLP problems. Gavin is now leading a team to leverage data to empower the most beloved Canadian coffee brand -- Tim Hortons by expediting the digital transformation and providing exceptional user experience.
Which talk track does this best fit into? Case Study
Who is this presentation for? Data Scientists/ ML Engineers
What you’ll learn: There is no other fast food company designing this multi-stage multi-task deep learning recommendation system for offer assignment.
Technical Level: 4
Are there any industries (in particular) that are relevant for this talk? Food & Beverages
Pre-requisite Knowledge: Deep learning general knowledge, VAE, collaborative filtering
What are the main core message (learning) you want attendees to take away from this talk? How to use deep learning to build multi tasking system to solve complicated recommendation problems, multi-task learning for recommendation systems
What is unique about this speech, from other speeches given on the topic? The recommender system has been designed by a lot of internet or tech companies, but their focus is always on click through rate. However, in our fast food industry, instead of just focusing on redemption rate for the offer recommendation, we also wanna achieve high incrementality when customer use the offer we recommended. This is really trick, since redemption rate and incrementality are always trade-off. Different from all the other companies especially inside of fast food industry, there is no good solution for this. We innovatively build multi-stage multi-task deep learning model to solve this problems perfectly.
Abstract: In the retail industry, digital transformation is an inevitable trend happening at a lot of big companies. Through the digital transformation, the brands could provide completely new user interface for users to improve the customer experience and company wise revenue at the same time. Offers is an commonly used strategy to increase the customer's stickiness to the brand and stretch the customers' spending. However, there are two obstacles ahead of us. First is that every week we only have limited spots to assign the offers to users, but the offers' pool is way bigger. Second, not like other internet company which mainly focus on customers' click rate on the recommendation, the retail business needs to focus on incremental sales the offer bring to us as well, but the incremental sales and redemption rate is a trade-off, so it is really hard to maintain perfect balance between both metrics. How to utilize the limit spots to maximize the recommended offer quality so that we could improve activation rate/ Redemption rate and revenue is the challenge we try to solve.
Can you suggest 2-3 topics for post-discussion? Recommender system, weekly offer in fast food industry
Unlocking the Power of Generative AI with Hugging Face Transformers
Philipp Schmid, Technical Lead, Hugging Face
About the Speaker: Philipp Schmid is a Technical Lead at Hugging Face with the mission to democratize good machine learning through open source and open science. Philipp is passionate about productionizing cutting-edge & generative AI machine learning models.
Which talk track does this best fit into? Workshop
Who is this presentation for? Senior Business Executives, Product Managers, Data Scientists/ ML Engineers and High-level Researchers, Product Managers, Data Scientists/ ML Engineers, ML Engineers, Researchers
What you’ll learn: Learn how to build AI-powered experiences leveraging the latest technology in Machine Learning and Generative AI with Hugging Face and Amazon SageMaker
Technical Level: 5
Are there any industries (in particular) that are relevant for this talk? Everyone.
Pre-requisite Knowledge: High level understanding of what Transformers are, but will be covered.
What are the main core message (learning) you want attendees to take away from this talk? Learn how to build AI-powered experiences leveraging the latest technology in Machine Learning and Generative AI with Hugging Face and Amazon SageMaker.
What is unique about this speech, from other speeches given on the topic? Deploying 10B+ parameter LMs is/was challenging and requires sometimes week of work without even optimizing the models thats going to be changed.
Abstract: Learn how to build AI-powered experiences leveraging the latest technology in Machine Learning and Generative AI with Hugging Face and Amazon SageMaker. Hugging Face has become the central hub for Machine Learning, with more than 100,000 free and accessible machine learning models to process and generate text, audio, speech, images and videos. We will discuss how over 15,000 companies are using Hugging Face to build AI into their applications, and participants will learn how they can do the same easily, leveraging Hugging Face open source models with the enterprise compliant environment of Amazon SageMaker.
Can you suggest 2-3 topics for post-discussion? Open-source vs closed-source, when to pretrain LLMs
Building a Customized, High-Performance Chat Engine with GPT-4
Ethan C. Jackson, Co-Founder and Research Lead, ChainML
Ron Bodkin, Co-founder and CEO, ChainML
Daniel Kur, Machine Learning Scientist, ChainML
About the Speaker: Ethan is co-founder and research lead at ChainML, a startup focused on building scalable, composable AI systems and applications. He is also a founding member of the Social AI Research Group at the University of Toronto. Ethan was previously an Applied ML Scientist with Vector Institute's AI Engineering Team, where he served as technical lead for several applied AI projects in collaboration with many industry and public sector partners. Ethan holds a PhD in Computer Science from Western University and trained as a postdoc under the supervision of Graham Taylor at the University of Guelph.
Ron is co-founder and CEO of ChainML, a startup that is delivering Generative AI supercharged by Web3. Ron was previously VP of AI Engineering and CIO at the Vector Institute and before that was responsible for Applied AI in the Google Cloud CTO office. Ron also was co-founder and CEO of enterprise AI startup Think Big Analytics that was acquired by Teradata and VP Engineering at AI pioneer Quantcast. Ron was also co-founder and CTO of C-Bridge Internet Solutions that IPO'd. Ron has a Master’s in Computer Science from MIT and an honors B.S. in Math and Computer Science from McGill University.
Daniel is a Machine Learning Scientist at ChainML, a startup that is delivering Generative AI supercharged by Web3. Prior to that, Daniel worked in the machine learning team at ServiceNow, joining as part of the acquisition of Element AI. Daniel also has experience in finance, starting his career in investment banking working on mergers and acquisitions at TD Securities. Daniel has a Master of Business Analytics and Bachelor of Business Administration from the Schulich School of Business at York University.
Which talk track does this best fit into? Workshop
Who is this presentation for? Senior Business Executives, Product Managers, Data Scientists/ ML Engineers and High-level Researchers, Product Managers
What you’ll learn: You'll learn how to apply an end-to-end experimental approach to building robust chat systems with GPT-4 at the core. We will go into details about the strenghts and limitations of both proprietary APIs and open-source frameworks, giving a pragmatic view on how to be productive with these tools today.
Technical Level: 5
Are there any industries (in particular) that are relevant for this talk? Banking & Financial Services, Information Technology & Service, Insurance, Marketing & Advertising, Telecommunications
Pre-requisite Knowledge:
What are ChatGPT and GPT-4? The difference between open and closed foundation models.
What are the main core message (learning) you want attendees to take away from this talk? How to use, stay up-to-date with Chat AI for business use cases
What is unique about this speech, from other speeches given on the topic? It is very specific about the challenges faced when integrating custom components into a generative AI chat system, like the ability to do database querying, data visualization, and forecasting. To do this in a high-performance, scalable way, you can't just delegate these to GPT Plugins. We will talk about how this is possible today with custom software, and how we think this will change as the ecosystem evolves.
Abstract: As business interest in generative AI continues to grow, it's crucial for developers to understand the technical aspects of integrating cutting-edge language models like GPT-4 into business applications with strong requirements for customization, performance, and reliability. In this hands-on workshop, we will present our reusable process for successful integration of GPT-4 into business applications. We will give details about our strategies for prompt engineering, context building, and response evaluation - all with the goal of helping LLMs to give their best possible answers, according to a diverse set of evaluation criteria. As a point of reference, we will share details about our experience developing a highly customized chat experience to the Space and Time Web3 Data Warehouse that includes special features like AI-assisted database querying, data visualization, and time series forecasting. We will also discuss our process for ongoing development and adaptation of chat applications, with a focus on how we are anticipating the rapid emergence of new tools and frameworks, particularly in the open-source domain. By the end of this workshop, participants will be able to simplify their approach to business chat application development by adopting or extending our process, and they will understand how development processes are likely to evolve with rapidly changing AI and LLM ecosystems.
Can you suggest 2-3 topics for post-discussion? Opportunities and limitations of building with open source LLMs, how to integrate with private data sources and/or proprietary tools, how small organizations can compete in the generative AI market.
Safely managing generative AI with humans-in-the-loop: lessons learned from risky real-world applications
Richard Zuroff, Senior Vice President Growth, Bluedot
Alexel Nordell-Markovits, Senior Vice President Growth, Moov AI
About the Speaker: Richard is the Senior Vice President of Growth at BlueDot, a global infectious disease intelligence company that uses human and artificial intelligence to detect and assess the risks of emerging and endemic disease threats. Richard began his career at McKinsey where he advised clients on advanced analytics, before founding the strategy and governance practice at Element AI, and continuing to work on democratizing the use of AI at DataRobot. Richard has spoken about the social and economic impact of AI/ML at the World Economic Forum, Partnership on AI, and Brookfield Institute. He is the author of a recent book chapter on Explainable AI for Financial Services, and has published in the Canadian Journal of Law and Technology. Richard holds an MBA, two Law degrees, and a BSc in Cognitive Science from McGill University.
Alexei has been building systems and teams within the context of modern AI for the better part of a decade. He has worked in diverse subfields, including process optimization, Mlops and Responsible AI. He is an AI director at Moov AI where he works with clients helping them discover and then implement AI solutions within business processes. In his spare time, he enjoys reading junky science-fiction.
Which talk track does this best fit into? Case Study
Who is this presentation for? Senior Business Executives, Product Managers, Data Scientists/ ML Engineers and High-level Researchers
What you’ll learn: Real-world case studies; insights from practitioners
Technical Level: 3
Are there any industries (in particular) that are relevant for this talk? Hospital & Health Care, Pharma and life sciences
Pre-requisite Knowledge: Basic awareness of language models
What is unique about this speech, from other speeches given on the topic? Challenges of making generative AI truthful with appropriate tone for a professional (not generic consumer-internet) setting has not been discussed much. And the application in relatively "high-risk" settings is unique
Abstract: Rapid progress in large language models (LLMs) has ignited interest in a wide array of exciting generative use cases for both consumer- and enterprise-facing applications. As adoption accelerates, it is critical to ensure these systems remain safe and trustworthy. Researchers are exploring technical solutions (such as constitutional AI) but in this talk we discuss two design patterns that involve putting humans-in-the-loop (HITL) of production-ready generative AI systems. HITL approaches to responsible generative AI are well aligned with regulators’ push for human oversight of “high risk” systems and are more likely to help companies create a strategic moat (compared to just using foundational models).
Can you suggest 2-3 topics for post-discussion? Responsible AI; Strategic differentiation in the era of foundational models; human-in-the-loop design patterns
The Natural Language Processing Revolution in Investment Management: Advice and Responsible Investing
Alik Sokolov, Co-Founder and CEO, Responsibli
Technical Level: 2
Are there any industries (in particular) that are relevant for this talk? Banking & Financial Services
Pre-requisite Knowledge: Some high level understanding of investment management; need not work in the industry
What are the main core message (learning) you want attendees to take away from this talk? The evolution in AI and Large Language Models will transform the field of finance, in some ways that are similar from other fields and in some ways that are quite unique. The attendees should be left with a better understanding of the potential of how AI can transform massive aspects of the industry like investment research, advice, and responsible investing.
What is unique about this speech, from other speeches given on the topic?I have a very unique mix of skills at the industry of machine learning, finance and academia. I have my CFA, work with some very prominent asset managers to help them integrate AI into their day-to-day process today, as well as do research on these topics as a University of Toronto graduate researcher.
Abstract: This talk will discuss the revolution of natural language processing in recent years, and how it applies to two diverse areas of investment management: investment advice, and the evolving landscape of responsible investing. Our ability to work with unstructured text data, which is abundant in investment management, has undergone several evolutions from the late 2010's: from sequence-to-sequence models for machine translation, to the advent of transformers and transfer learning, to the recent breakthroughs achieved by Large Language Models like Chat GPT. These changes will have a profound impact on investment management, and we will examine two case studies in investment management and responsible investing. We will also examine their long-term future, and how the technical approaches to both are likely to intersect.
Can you suggest 2-3 topics for post-discussion? Large Language Models - what has changed, Human &a
Where is it happening?
The Carlu, 444 Yonge St, Toronto, CanadaEvent Location & Nearby Stays:
CAD 0.00 to CAD 570.00
