Prof. Maria De Iorio - Bayesian Mixture Models: Foundations and Innovations
Schedule
Fri Feb 14 2025 at 02:00 pm to 03:00 pm
UTC+00:00Location
Emmeline Suite Open, Christabel Pankhurst Building | Manchester, EN
About this Event
The University of Manchester is hosting a hybrid seminar with Professor Maria De Iorio (National University of Singapore).
Location: Emmeline Suite Open, Christabel Pankhurst Building & online.
Bayesian Mixture Models: Foundations and Innovations
Abstract
Mixture models are essential tools for analysing heterogeneous populations. From a Bayesian nonparametric perspective, we introduce a novel class of priors, the Normalised Independent Point Process, and develop both marginal and conditional algorithms for finite mixture models with a random number of components. By employing an auxiliary variable MCMC approach, we efficiently address the challenges posed by intractable posterior distributions, creating a flexible and extensible framework for Bayesian modelling.
In this talk, we highlight key extensions of this framework for the analysis of multiview data, where dependencies arise across multiple data domains. To address these complexities, we propose a probabilistic framework for conditional partial exchangeability, specifically designed for multiview and longitudinal data. This framework introduces flexible random partitions that vary across features, effectively capturing dependencies across multiple domains. Furthermore, it can be extended to link random partitions of subjects across datasets through an underlying shared partition structure, enabling information sharing and facilitating robust inference. By accounting for within-subject dependencies and modelling marginal relationships between datasets, this approach enhances both the flexibility and interpretability of clustering structures.
We also present Bayesian Distance Clustering (BDC), a hybrid approach that defines the likelihood on pairwise distances between observations rather than on the observations themselves. The novelty of BDC lies in its ability to incorporate both cohesion and repulsion terms, ensuring cluster identifiability. This method strikes a balance between computational feasibility and probabilistic interpretability, making it particularly effective for clustering large and complex datasets. We extend BDC to multiview data by introducing scalable models that maintain predictive accuracy while improving efficiency. Inspired by K-medoids, we propose a novel tessellation-based method that identifies tessellation centres, or "medoids," significantly enhancing the efficiency of the clustering process.
We validate these methods through extensive simulations and applications to real-world datasets, demonstrating their effectiveness across a variety of contexts. These advancements provide a robust and versatile toolkit for addressing modern data analysis challenges, particularly in the context of multiview and longitudinal data.
This event is supported by the AI Hub for Causality in Healthcare AI with Real Data (CHAI), of which Manchester is a spoke. CHAI unites an international consortium of universities, industry partners, government bodies, and regulatory entities to develop cutting-edge innovations to enhance patient care and outcomes.
Where is it happening?
Emmeline Suite Open, Christabel Pankhurst Building, Dover Street, Manchester, United KingdomEvent Location & Nearby Stays:
USD 0.00
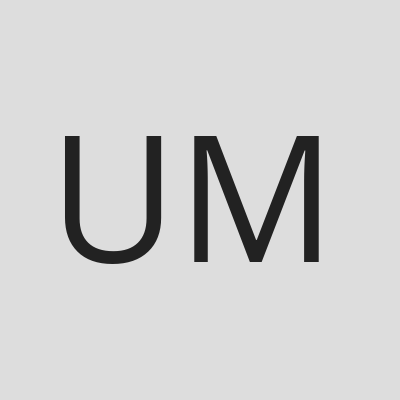